The last months, due to Covid-19 factories, offices and institutions have been closed or been working on less power. Now, factories and offices want to open again. In the meantime, it is clear that Covid-19 will be with us for a long time. Employers want a safe as possible environment for their employees and visitors, as well as healthcare institutions and tourist and cultural providers. In addition, companies have a legal obligation to ensure a safe environment. And to take measures where necessary, so that the social distance is respected.
The Social Distance Badge is the device with which the 1.5-meter distance can be maintained. Simple, safe and without privacy issues
Work and recreation in times of Corona
For whom, what, why?
- Factories
- Construction and installation sites
- Logistics: ports, warehouses
- Transport (train, airports, busses)
- Offices and Facility management
- Healthcare
- Touristic, sports and cultural sites and events
Factories
Factories and offices want to open again. Where offices can still choose by letting employees work from home, factories do not have this luxury. At assembly lines, many people are working together, concentrated to carry out their work. Then the 1.5 meter social distance may be ignored.
Construction and installations, logistics and transport
Employees are also unable to work from home during construction and installations. Many employees work side by side and close together. The employee will often need his focus to perform his work accurately and pay attention to other forms of safety, for example to prevent him from slipping or falling down. Employees can then forget to maintain the 1.5 meter social distance. The Social Distance badge helps to remind the employee to keep distance.
Offices and facility management
In the last months, many employees of offices have worked at home. But for social bonding and the well-being of employees, it’s important that employees are regularly present in the office. And not every office has the option to offer working from home.
Employees walk from workplace to company restaurant and meeting and back again. And meanwhile, facility managers walk through the entire building. Then it is important to keep the 1.5-meter distance, since 1 employee can spread the Corona-19 virus in the entire building.
Healthcare
For some groups, Covid-19 is particularly dangerous: the elderly and people who already have a condition. It is important for them that social distance is maintained. By clients, staff and visitors. In addition, some residents must be reminded to keep 1.5 meters.
Tourist, Cultural, Recreational, Sports sites and events
Visitors get mixed up, are enthusiastic and forget to keep their distance. Or are there visitors who do not take the 1.5 meter into account and thus pose a risk. With the Social Distance Badge, you can safely open again.
Guaranteed 1,5 meters distance
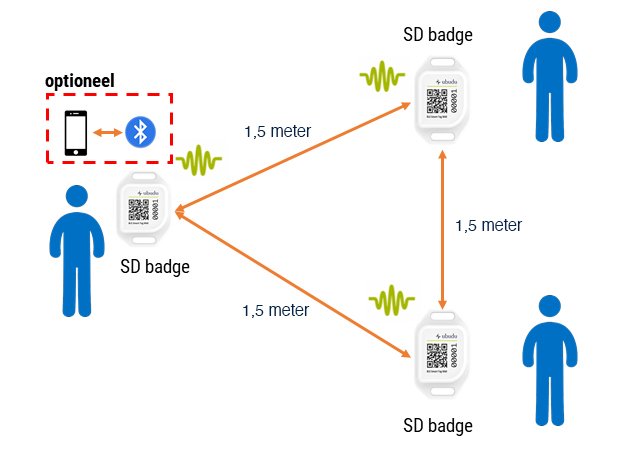
All employees (and customers or visitors) receive the SD badge upon entrance. This is worn around the wrist or neck. The SD Badge continuously scans its environment for other badges via a so-called Ultra Wide Band signal. This signal provides the most accurate distance measurement. As soon as 2 (or more) badges are less than 1.5 meters apart, they give a warning sound and/ or light signal. This allows everyone to keep enough distance. As soon as there are another safe 1.5 meters, the signal will stop.
Read more and order now: https://covidbuzzer.com