Posts
In ECG signal processing, the Removal of 50/60Hz powerline interference from delicate information rich ECG biomedical waveforms is a challenging task! The challenge is further complicated by adjusting for the effects of EMG, such as a patient limb/torso movement or even breathing. A traditional approach adopted by many is to use a 2nd order IIR notch filter:
\(\displaystyle H(z)=\frac{1-2cosw_oz^{-1}+z^{-2}}{1-2rcosw_oz^{-1}+r^2z^{-2}}\)
where, \(w_o=\frac{2\pi f_o}{fs}\) controls the centre frequency, \(f_o\) of the notch, and \(r=1-\frac{\pi BW}{fs}\) controls the bandwidth (-3dB point) of the notch.
What’s the challenge?
As seen above, \(H(z) \) is simple to implement, but the difficulty lies in finding an optimal value of \(r\), as a desirable sharp notch means that the poles are close to unit circle (see right).
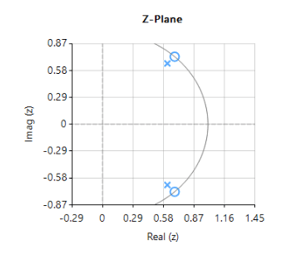
In the presence of stationary interference, e.g. the patient is absolutely still and effects of breathing on the sensor data are minimal this may not be a problem.
However, when considering the effects of EMG on the captured waveform (a much more realistic situation), the IIR filter’s feedback (poles) causes ringing on the filtered waveform, as illustrated below:
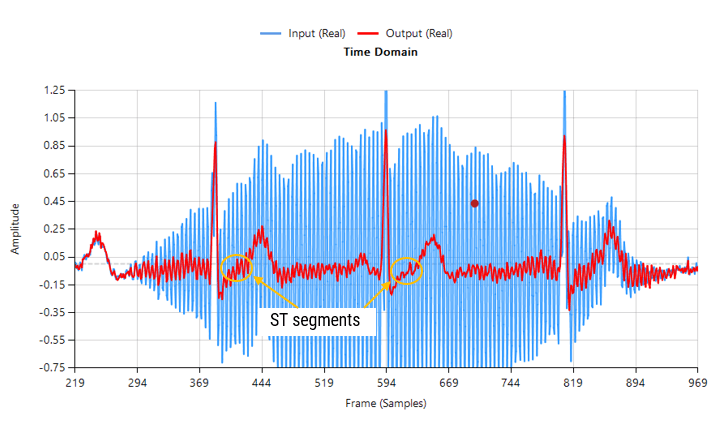
Contaminated ECG with non-stationary 50Hz powerline interference (IIR filtering)
As seen above, although a majority of the 50Hz powerline interference has been removed, there is still significant ringing around the main peaks (filtered output shown in red). This ringing is undesirable for many biomedical applications, as vital cardiac information such as the ST segment cannot be clearly analysed.
The frequency reponse of the IIR used to filter the above ECG data is shown below.
IIR notch filter frequency response
Analysing the plot it can be seen that the filter’s group delay (or average delay) is non-linear but almost zero in the passbands, which means no distortion. The group delay at 50Hz rises to 15 samples, which is the source of the ringing – where the closer to poles are to unit circle the greater the group delay.
ASN FilterScript offers designers the notch() function, which is a direct implemention of H(z), as shown below:
ClearH1; // clear primary filter from cascade ShowH2DM; // show DM on chart interface BW={0.1,10,.1,1}; Main() F=50; Hd=notch(F,BW,"symbolic"); Num = getnum(Hd); // define numerator coefficients Den = getden(Hd); // define denominator coefficients Gain = getgain(Hd); // define gain
Savitzky-Golay FIR filters
A solution to the aforementioned mentioned ringing as well as noise reduction can be achieved by virtue of a Savitzky-Golay lowpass smoothing filter. These filters are FIR filters, and thus have no feedback coefficients and no ringing!
Savitzky-Golay (polynomial) smoothing filters or least-squares smoothing filters are generalizations of the FIR average filter that can better preserve the high-frequency content of the desired signal, at the expense of not removing as much noise as an FIR average. The particular formulation of Savitzky-Golay filters preserves various moment orders better than other smoothing methods, which tend to preserve peak widths and heights better than Savitzky-Golay. As such, Savitzky-Golay filters are very suitable for biomedical data, such as ECG datasets.
Eliminating the 50Hz powerline component
Designing an 18th order Savitzky-Golay filter with a 4th order polynomial fit (see the example code below), we obtain an FIR filter with a zero distribution as shown on the right. However, as we wish to eliminate the 50Hz component completely, the tool’s P-Z editor can be used to nudge a zero pair (shown in green) to exactly 50Hz.
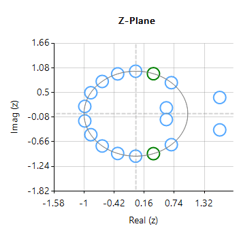
The resulting frequency response is shown below, where it can be seen that there is notch at exactly 50Hz, and the group delay of 9 samples (shown in purple) is constant across the frequency band.
FIR Savitzky-Golay filter frequency response
Passing the tainted ECG dataset through our tweaked Savitzky-Golay filter, and adjusting for the group delay we obtain:
Contaminated ECG with non-stationary 50Hz powerline interference (FIR filtering)
As seen, there are no signs of ringing and the ST segments are now clearly visible for analysis. Notice also how the filter (shown in red) has reduced the measurement noise, emphasising the practicality of Savitzky-Golay filter’s for biomedical signal processing.
A Savitzky-Golay may be designed and optimised in ASN FilterScript via the savgolay() function, as follows:
ClearH1; // clear primary filter from cascade interface L = {2, 50,2,24}; interface P = {2, 10,1,4}; Main() Hd=savgolay(L,P,"numeric"); // Design Savitzky-Golay lowpass Num=getnum(Hd); Den={1}; Gain=getgain(Hd);
Deployment
This filter may now be deployed to variety of domains via the tool’s automatic code generator, enabling rapid deployment in Matlab, Python and embedded Arm Cortex-M devices.
Author
-
Sanjeev is an AIoT visionary and expert in signals and systems with a track record of successfully developing over 25 commercial products. He is a Distinguished Arm Ambassador and advises top international blue chip companies on their AIoT solutions and strategies for I4.0, telemedicine, smart healthcare, smart grids and smart buildings.
View all posts
For many IoT sensor measurement applications, an IIR or FIR filter is just one of the many components needed for an algorithm. This could be a powerline interference canceller for a biomedical application or even a simpler DC loadcell filter. In many cases, it is necessary to integrate a filter into a complete algorithm in another domain. ASN Filter Designer’s automatic code generator greatly simlifies exporting to Python.
Python is a very popular general-purpose programming language with support for numerical computing, allowing for the design of algorithms and performing data analysis. The language’s numpy
and signal
add-on modules attempt to bridge the gap between numerical algorithmic languages, such as Matlab and more traditional programming languages, such as C/C++. As such, it is much more appealing to experienced programmers, who are used to C/C++ data types, syntax and functionality, rather than Matlab’s scripting language that is more aimed at mathematicans developing algorithmic concepts.
ASN Filter Designer automatic code generator for Python
The ASN Filter Designer greatly simplifies exporting a designed filter to Python via its automatic code generator. The code generator supports all aspects of the ASN Filter Designer, allowing for a complete design comprised of H1, H2 and H3 filters and math operators to be fully integrated with an algorithm in Python.
The Python code generator can be accessed via the filter summary options (as shown on the right). Selecting this option will automatically generate a Python .py
design file based on the current design settings.
Version 5 of the tool has a completely revamped filter summary UI, and now includes built in AI to analyse the filter cascade for any potential problems.
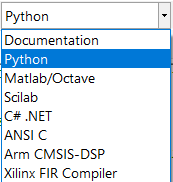
The project wizard bundles all of the necessary SDK framework files needed to run the designed filter cascade without the need for any other dependencies or 3rd party plugins.
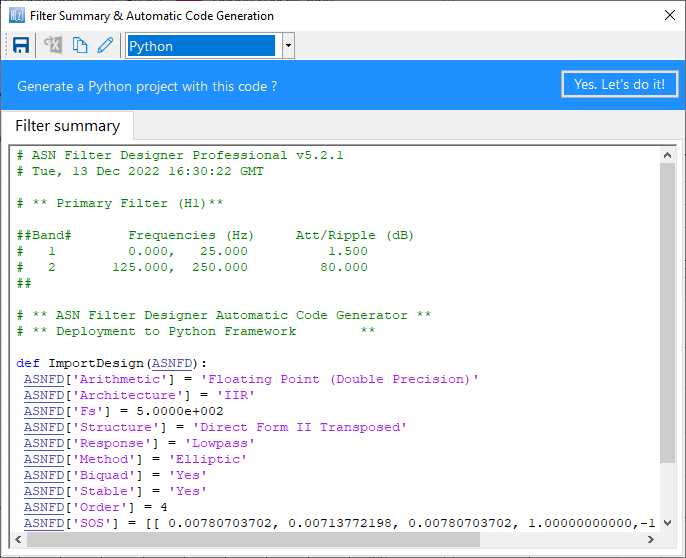
The framework supports both Real and Complex filters in floating point only, and is built on ASN IP blocks, rather than Python’s signal
module, which was seen to struggle with managing complex data. Thus, in order to expedite algorithm development with the framework, the following three demos are provided:
ASNFDPythonDemo
: main demo file with various examplesRMSmeterDemo
: An RMS amplitude powerline meter demoEMGDataDemo
: An EMG biomedical demo with a HPF, 50Hz notch filter and averaging
An example of the summary of all of generated files (including the framework files) is shown below.
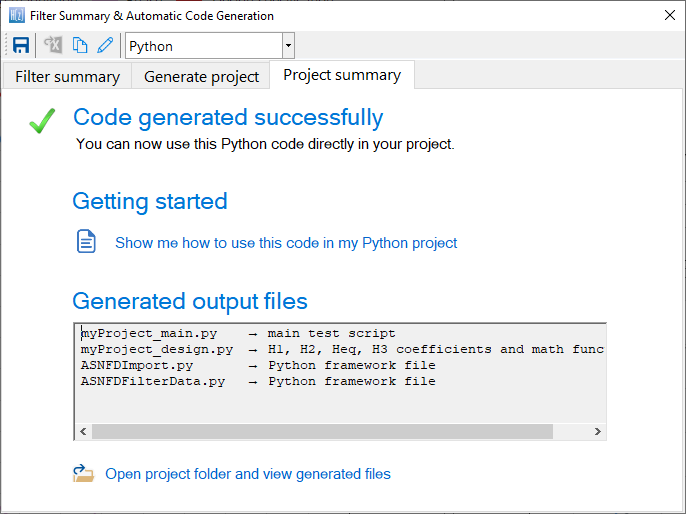
These files can be used directly in your Python project.
Did you know that there are 23 billion IoT embedded devices currently deployed around the world? This figure is expected to grow to a whopping 1 trillion devices by 2050!
Less known, is that 80% of IoT devices are based around Arm’s Cortex-M microcontroller technology. Sometimes clients ask us if we support their Arm Cortex-M based demo-board of choice. The answer is simply: yes!
200+ IC vendors supported
The ASN Filter Designer has an automatic code generator for Arm Cortex-M cores, which means that we support virtually every Arm based demo-board: ST, Cypress, NXP, Analog Devices, TI, Microchip/Atmel and over 200+ other manufacturers. Our compatibility with Arm’s free CMSIS-DSP software framework removes the frustration of implementing complicated digital filters in your IoT application – leaving you with code that is optimal for Cortex-M devices and that works 100% of the time.
The Arm Cortex-M family of microcontrollers are an excellent match for IoT applications. Some of the advantages include:
- Low power and cost – essential for IoT devices
- Microcontroller with DSP functionality all-in-one
- Embedded hardware security functionality
- Cortex-M4 and M7 cores with hardware floating support (enhanced microcontrollers)
- Freely available CMSIS-DSP C library: supporting over 60 signal processing functions
Automatic code generation for Arm’s CMSIS-DSP software framework
Simply load your sensor data into the ASN Filter Designer signal analyser and perform a detailed analysis. After identifying the wanted and unwanted components of your signal, design a filter and test the performance in real-time on your test data. Export the designed design to Arm MDK, C/C++ or integrate the filter into your algorithm in another domain, such as in Matlab, Python, Scilab or Labview.
Use the tool in your RAD (rapid application development) process, by taking advantage of the automatic code generation to Arm’s CMSIS-DSP software framework, and quickly integrate the DSP filter code into your main application code.
Let the tool analyse your design, and automatically generate fully compliant code for either the M0, M0+, M3, M4 and the newer M23 and M33 Cortex cores. Deploy your design within minutes rather than hours.
Proud Arm knowledge partner
We are proud that we are an Arm knowledge partner! As an Arm DSP knowledge partner, we will be kept informed of their product roadmap and progress for the coming years.
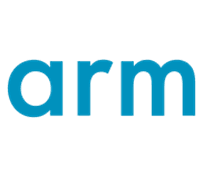
Try it for yourself and see the benefits that the ASN Filter Designer can offer your organisation by cutting your development costs by up to 75%!
Advanced Solutions Nederland B.V.
Lipperkerstraat 146
751DD Enschede
The Netherlands
Tel: +31 652460840
General enquiries: info@advsolned.com
Technical support: support@advsolned.com
Sales enquiries: sales@advsolned.com