DSP voor ingenieurs: de ASN Filter Designer is de ideale tool om de sensordata snel te analyseren en te filteren. Maak een algoritme binnen enkele uren in plaats van dagen. Wanneer u met sensorgegevens werkt, herkent u deze uitdagingen waarschijnlijk:
- Mijn sensordatasignalen zijn te zwak om zelfs maar een analyse te maken. Daarom heb ik versterking van de signalen nodig
- Waar ik een vlakke lijn zou verwachten, zien de gegevens eruit als een puinhoop door interferentie en andere vervuiling. Ik moet de gegevens eerst opschonen voordat ik ze analyseer.
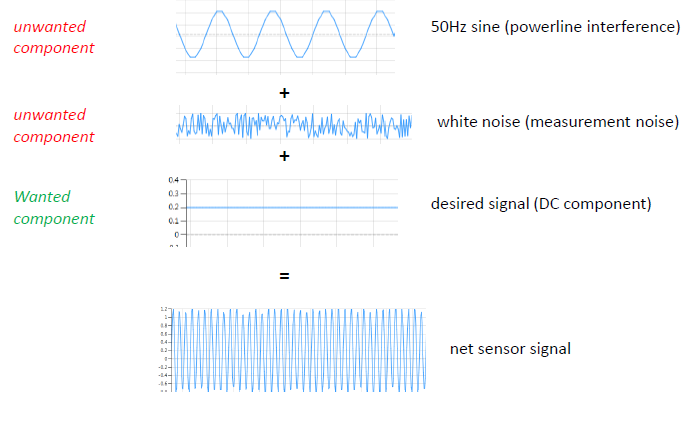
Waarschijnlijk heb je tot nu toe dagen of zelfs weken gewerkt aan signaalanalyse en filtering. Het ontwikkelingstraject is over het algemeen langzaam en zeer pijnlijk. Denk maar eens aan het aantal uren dat je had kunnen besparen als je een ontwerptool had gehad die alle algoritmische details voor jou beheerde. ASN Filter Designer is een standaardoplossing voor de industrie die wordt gebruikt door duizenden professionele ontwikkelaars die wereldwijd aan iot-projecten werken.
Onze nauwe samenwerking met Arm en ST zorgt ervoor dat alle ontworpen filters 100% compatibel zijn met alle Arm Cortex-M processoren, zoals de populaire STM32-familie van ST.
Uitdagingen voor ingenieurs
- 90% van IoT smart sensors zijn gebaseerd op Arm Cortex-M processor technologie
- Sensor signal processing is moeilijk
- Sensoren hebben moeite met interferentie en allerlei ongewenste componenten
- Hoe ontwerp ik een filter dat voldoet aan mijn requirements?
- Hoe kan ik mijn ontworpen filter controleren op testdata?
- Voor betere product performance is schone sensor data nodig
- Tijdrovend proces om een filter op een embedded processor te implementeren
- Tijd is geld!
Ontwerpers verzanden vaak met traditionele tooling. Deze vereist meestal een iteratieve, trial and error aanpak of deskundige kennis. Met deze aanpak gaat kostbare tijd verloren. ASN Filter Designer helpt u met een interactieve ontwerpmethode. Hierbij voert de tool automatisch de technische specificaties in op basis van eisen die de gebruiker grafisch heeft ingevoerd.
Snelle ontwikkeling van het DSP-algoritme
- Volledig gevalideerd filterontwerp: geschikt voor toepassing in DSP, Arm microcontroller, FPGA, ASIC of PC-toepassing
- Automatische gedetailleerde ontwerpdocumentatie: de Filter Designer helpt je met documenatie, waardoor je de peer review kunt versnellen en projectrisico’s verlaagt
- Eenvoudige overdracht: projectdossier, documentatie en testresultaten bieden een gemakkelijk manier voor overdracht aan collega’s of andere teams
- Gemakkelijk in te passen in nieuwe scenario’s: het ontwerp kan eenvoudig worden aangepast aan andere eisen en scenario’s, zoals 60Hz interferentieonderdrukking op de voedingslijn, in plaats van de Europese 50Hz.
ASN Filter Designer: de snelle en intuitieve filter designer
De ASN Filter Designer is het ideale hulpmiddel om sensorgegevens snel te analyseren en filteren. Indien nodig kun je jouw gegevens eenvoudig naar tools als Matlab en Python exporteren voor verdere analyse. Daarom is het ideaal voor ingenieurs die een krachtige tool voor signaalanalyse nodig hebben en een datafilter voor hun IOT-toepassing moeten maken. Zeker als je af en toe een datafilter moet maken. Vergeleken met andere tools creeer je een algoritme binnen enkele uren in plaats van dagen.
Exporteer jouw algoritmes naar Matlab, Python of een Arm microcontroller
Je kunt veel tijd besparen doordat je met ASN Filter Designer algoritmes eenvoudig kunt implementeren in Matlab, Python of direct op een Arm-microcontroller omdat de Filter Designer automatisch code generateert.
Onmiddelijke verlichting
Denk eens aan het aantal uren dat je had kunnen besparen als je een ontwerptool had gehad die alle algoritmische details voor je beheerde.
ASN Filter Designer is een standaardoplossing in de sector die wordt gebruikt door duizenden professionele ontwikkelaars die wereldwijd aan ivd-projecten werken. Onze nauwe samenwerking met Arm en ST zorgt ervoor dat alle filters 100% compatibel zijn met alle Arm Cortex-M processoren.
Hoeveel pijnverzachting kun je voor 145 Euro kopen?
Omdat veel technici onze ASN Filterontwerper voor korte tijd nodig hebben, is een licentie van 145 euro voor slechts 3 maanden mogelijk!
Vraag jezelf maar af: is 145 Euro een eerlijke prijs om te betalen voor onmiddellijke pijnverlichting en resultaat? Wij denken van wel. Bovendien hebben we een licentie voor 1 jaar en zelfs een eeuwigdurende licentie. Download de demo om het zelf te zien of neem contact met ons op voor meer informatie